At UBalt, we believe that AI is not a threat, but an opportunity. Under the direction of the Center for Excellence in Learning, Teaching, and Technology (CELTT), the University is ensuring that the technology complements education rather than overshadowing it.
BY POORNIMA APTE
ILLUSTRATIONS BY MARIO WAGNER
For a long time, higher education has moved at a predictable pace with educators adopting new technology tools gradually. The advent of artificial intelligence (AI) feels much more disruptive, especially because its pace of evolution is so rapid.
Since news of the impressive capabilities of generative AI—ChatGPT is its most familiar variation—broke in November 2022, the technology has been making systematic inroads in higher education. A 2024 Turnitin survey of college students in the fall 2023 term found that more than half used generative AI in some manner.
The post-AI landscape is entirely new—and unprecedented. With respect to AI, “what I would offer is that The University of Baltimore, as other universities, is in the midst of what might be similar to the Cambrian explosion in prehistory, when you had so many life forms in such a small time and such accelerated evolution—when you’re in the middle of something that dynamic, it’s unclear where you are relative to others,” said Dr. Alan Lyles, Henry A. Rosenberg Professor of Government, Business and Nonprofit Partnerships at the College of Public Affairs.
“Educators are having to make choices that are strategic, tactical, operational. They are committing resources and setting priorities perhaps for a decade ahead, but those decisions have immediate consequences,” Lyles said. “They’ve never done any of this.”
Julia Goffredi, instructional designer & emerging technology lead at the Bank of America Center for Excellence in Learning, Teaching and Technology (CELTT) agrees. The challenge, she said, is “how do you make meaning of something that’s so radically different from the way you’re used to presenting and disseminating information and knowledge?”
AI STRATEGY UNDER CELTT LEADERSHIP
Making meaning of this disruption is a challenge that CELTT is tackling head on under the leadership of its director of teaching and learning excellence, Dr. Jessica Stansbury.
A doctorate in instructional technology had already equipped Stansbury with the tools for integrating new technology into classrooms. When she first test drove the public-facing version of ChatGPT, she was excited about its potential to help faculty. “My first thought was like, this is great for efficiency; faculty would be able to get rid of some of the tedious and mundane tasks they do,” Stansbury said.
But when not all educators seemed excited—some were worried or skeptical about the technology’s ramifications—Stansbury knew CELTT had a more complex challenge on its hands. The problem: How to square faculty concerns with the University’s motto, “Knowledge that Works.”
Stansbury’s views on the acquisition of knowledge—“we are facilitators, not gatekeepers”— shapes CELTT’s approach to AI at UBalt.
“When you tie it back to [the University’s] vision, our goal is to make sure our students are prepared to navigate a modern workplace,” Goffredi said. And that modern workplace will need a knowledge of AI tools. “We’re setting up our students for success in society, so we have to recognize that AI is not going away and it’s going to be an AI world,” Stansbury said.
LAYING THE GROUNDWORK FOR AI INTEGRATION
Setting up students for success starts with the basic building blocks of AI integration, and UBalt is making a conscious—and intentional—effort to approach this technology proactively rather than reactively.
According to Stansbury, campus partners “will assess the immediate and emerging AI applications most likely to impact teaching, learning and research and explore the long-term needs of institutions, instructors and scholars, as they navigate this environment.”
Among the first orders of business: joining the “Making AI Generative for Higher Education” initiative, launched by Ithaka S+R, a higher-education research firm. Along with 17 other member universities in its cohort, UBalt is in the midst of a four-phase, two-year research project that aims to establish the responsible use of generative AI on campus. Being part of the Ithaka project enables UBalt to observe how peers are faring and to establish guidelines and guardrails for AI use. While the culmination of the Ithaka project will be a concrete set of guidelines for the entire University to follow, CELTT has begun the work of developing guidelines for AI use now.
Understanding that not all members of the campus will adopt the technology at the same pace, CELTT has opted against a top-down AI policy. UBalt has instead taken a data-driven approach and has regularly polled its community and brought them along on the AI adoption journey. “Just as we wouldn’t administer medicine without clinical trials, AI policies should not be created without the expertise of educators and researchers, with all stakeholder voices in the conversation,” Stansbury has written.
To introduce faculty to AI, Stansbury arranged a “learn with me” session for instructors in January 2023, just a few months after ChatGPT’s public unveiling. After encouraging casual conversations about AI in early 2023, it was time for a more formal introduction.
CELTT recruited Amr Kadry, M.S. ’20, coordinator of tutoring and academic coaching services at UBalt’s Robert L. Bogomolny Library to develop courses for both faculty and students. Kadry applied for and was awarded an Elkins SoTL (Scholarship for Teaching and Learning) 2023-24 fellowship, granted by the University System of Maryland’s William E. Kirwan Center for Academic Innovation, to design and research the impact of these courses.
The asynchronous courses, called From Chalkboards to Chatbots, introduce the University community—including students and faculty—to the basics, which they can visit at their own pace. The gentle (yet structured) start was intentional. “We’re not fully pushing the tools, and at the same time, we’re removing some of the fearmongering. The main purpose is to just expose everyone to AI and start the conversation,” Kadry said.
At the same time, said Goffredi, CELTT’s approach to AI literacy has been one that “dissipates the fear but keeps the curiosity. We didn’t want folks to just jump off the cliff. We need to make sure that everything’s tight and secure before you go bungee jumping.”
Also key has been the focus on developing AI literacy skills rather than expertise with a single platform, Kadry said. Such an approach is a reminder that the platforms might evolve—Bard today, Gemini tomorrow—but the fundamental technology behind AI is what educators and students will need to grasp. “We’re taking more of a mindset that it’s important for us to start integrating AI literacy into the UBalt educational experience,” Kadry said.
AI FOR EDUCATORS
University educators are also doing their part to integrate AI into the UBalt experience. They have participated in the CELTT courses and been active and vocal in shaping how the University approaches the technology.
“Being proficient in the use and the education of AI as a tool is the price of admission to being a professor today. Period. Hard stop,” Lyles said.
A few educators have also drafted policies for the technology’s use in their classrooms. “If there’s no AI policy then each educator is on their own and we’re unnecessarily introducing entropy,” Lyles said. To avoid the problem, he decided to craft an AI policy, which itself would be refined over the semesters. “I’m going to incorporate feedback as we go along but it gives learners a knowable target,” Lyles said. “It gives guardrails and also aligns with assignments and expectations.”
“Being proficient in the use and the education of AI as a tool is the price of admission to being a professor today. Period. Hard stop.”
DR. ALAN LYLES
It’s not just about policy for student AI use. Having generative AI has forced educators to rethink assessments and how to measure what students are actually learning. “I was kind of happy when AI came along because it really shook up how we think about teaching,” Stansbury said. “If students can finish an assignment using just AI, then it’s probably not a very good assessment of learning.”
“The challenge for faculty is that for them to reimagine assignments, they have to have some knowledge of ChatGPT’s abilities and how it works,” said Dr. William Carter, associate professor of management in the Merrick School of Business and recipient of the Yale Gordon Distinguished Teaching Professorship. It says something that Carter, who usually guides companies on how to carefully navigate new technologies, is now so invested in a disruptive technology much closer to home. In early semesters, he recommended students use generative AI for extra-credit assignments; he’s now moving to incorporate it directly into his curriculum.
Lyles uses Wolfram|Alpha, a large language model based on mathematics and logics, as a way of having students take over the generative AI reins. Home assignments include using Wolfram for part of the work and understanding what the model can (and cannot) do.
Educators are also using generative AI as assistants for their own work, a result that Stansbury had first visualized. “What’s also interesting to me as a faculty member is that it makes it easier for me to create my own course content, to better design exercises and assignments,” Carter said.
AI FOR STUDENTS
The worries that students will use generative AI tools like ChatGPT to cheat are valid but not justified, according to Stansbury. “Most students that come to college, they want to learn, they want to be educated, they don’t want to cheat, they’re paying to go to school,” Stansbury said.
Lyles cautions against running with a one-size-fits-all approach to AI policy for students. The focus on the humanistic should not be lost in an attempt to embrace tech, according to Lyles. “From a teaching perspective, we have learners who are often non-traditional. Some are coming back to school as master’s students or undergraduates who didn’t come directly out of high school,” Lyles said.
Jay Knight, clinical law professor at UBalt, also wants students to take AI with a grain of salt. He reminds them about AI’s shortcomings: the answers are not always accurate. Students have to verify them and not accept them as blind facts. Second, using generative AI is not a private endeavor; models chew up the data you feed them. Finally, answers are not consistent—what you receive today might not be the same as one tomorrow.
“We’re setting up our students for success in society, so we have to recognize that AI is not going away and it’s going to be an AI world.”
DR. JESSICA STANSBURY
Knight teaches students to use generative AI to improve the tone and tenor of their communications with clients. It’s especially useful, he noted, for instances where writing is not a strength for students, especially those from underrepresented backgrounds.
The question of equity in access to generative AI tools has also surfaced and is an important one to address. “AI is just a tool, and humans get to decide how it’s deployed, so let’s deploy it for good and help level the playing field for a lot of students, especially from minority and underrepresented backgrounds,” Stansbury said.
FUTURE DIRECTION
The data-informed approach to AI adoption is already moving the needle at UBalt, especially by solidifying the University’s thought leadership in advancing AI in education and sparking dialogue within and beyond the campus community.
The University of Baltimore just hosted its first Generative AI Summit (p. 18), bringing faculty and students together with local community and industry leaders over three days in June to explore how AI can be integrated into various academic disciplines to drive innovation and excellence.
In September, UBalt joined forces with the University of Maryland, Baltimore County, and Johns Hopkins University to launch AI in Practice, a monthly webinar series dedicated to discussing the evolving role of Artificial Intelligence in teaching and the essential skills students need to thrive in an AI-enhanced workforce.
And on campus, Stansbury is already looking ahead to working with UBalt’s Division of Academic Affairs as they collaboratively develop a concrete set of guidelines for faculty related to the adoption of AI, just one of many planned initiatives based on the results of her team’s work with the Ithaka project.
In the meantime, CELTT continues to steer the AI conversation, navigating choppy waters with both enthusiasm and restraint, a tough balance to strike, educators say.
“We have to keep learning about AI. It’s going to continue to grow, and we can’t just stick our heads in the sand and act like it’s not going to happen,” Stansbury said. “With our approach we’re giving students autonomy over their learning, and that’s the way to create lifelong learners.”
AI TERMINOLOGY GLOSSARY
In the rapidly evolving field of artificial intelligence, understanding key concepts is crucial for grasping the broader landscape and applications, particularly in generative AI. While there are countless terms and nuances within AI, this glossary highlights 14 fundamental concepts that are essential for anyone engaging with generative AI in an educational setting. These terms provide a foundational understanding for navigating the complexities of this technology.
Please keep in mind that this glossary covers the core terms necessary for a foundational understanding of generative AI and is not an exhaustive list of AI terminology.
Artificial Intelligence (AI):
The overarching field focused on creating systems that can simulate human-like intelligence, such as learning, reasoning, problemsolving and perception.
Machine Learning (ML):
A subset of AI that involves algorithms that allow computers to learn from data and improve their performance over time without being explicitly programmed.
Neural Networks:
Computational models inspired by the human brain, consisting of layers of interconnected nodes (neurons). Neural networks are the foundation of many ML and deep learning models, enabling complex pattern recognition.
Deep Learning:
A specialized area within ML that uses neural networks with many layers (deep neural networks). It is particularly effective for processing and learning from large datasets, often used in tasks like image and speech recognition.
Generative AI (sometimes seen as GAI or GenAI):
A subset of deep learning that focuses on creating new content, such as text, images or audio. Generative AI models are trained on extensive datasets to generate outputs that resemble real-world examples.
Large Language Model (LLM):
A type of AI model trained on vast amounts of text data to understand and generate human language.
Natural Language Processing (NLP):
A branch of AI and ML that deals with the interaction between computers and human language. NLP involves understanding, interpreting and generating human language and is used in applications like language translation, sentiment analysis and dialogue systems.
Vector Search:
A technique used in AI and NLP to find and compare items based on their features. Each item is represented as a vector, similar to a point in a multi-dimensional space. This method helps identify similar items or content quickly, often using ML models to process the data.
Chatbots:
AI-driven systems that use NLP to simulate human conversation. They can answer questions, provide information and perform tasks based on user input, and are commonly used in customer service and educational settings.
Training Data:
The dataset used to train AI models, including those in ML, deep learning and generative AI. The quality and diversity of training data are critical for developing accurate and unbiased models.
Prompt Engineering:
The practice of designing inputs (prompts) to guide the outputs of generative AI models. This technique is crucial for achieving specific and relevant results, especially in text generation applications.
Hallucinations:
A phenomenon in generative AI and NLP where models produce outputs that are not based on actual data or logical reasoning. These outputs can be incorrect, nonsensical or entirely fabricated.
Bias:
Refers to systematic errors or prejudices in AI model predictions or outputs, often stemming from the training data or algorithmic design. Addressing bias is essential to ensure fairness and accuracy in AI systems.
AI Literacy:
The knowledge and skills required to understand, use and critically evaluate AI technologies. AI literacy involves understanding the basics of AI, ML, deep learning and generative AI, as well as the ethical and societal implications of these technologies
FROM CHALKBOARDS TO CHATBOTS
Unlocking and Exploring the Potential of AI in Higher Learning
Developed at the Bank of America Center for Excellence in Learning, Teaching and Technology, this 5-module course offers undergraduate and graduate students at the University of Baltimore an in-depth exploration of generative AI’s impact on higher education. It covers AI in education, ethics, plagiarism prevention, limitations and effective strategies through reflections and hands-on activities across five self-paced modules.
Now available online for free, you can browse course materials and learning resources, interact with generative AI tools and use self-guided reflections to consider the impact AI has had in your own life, personally or professionally.
From Chalkboards to Chatbots
Student Edition, Fall 2024
Simple Book Publishing
ubalt.pressbooks.pub/fctcstudent
TYPES OF GENERATIVE AI TOOLS
Generative AI tools include a variety of apps, platforms and plugins that use machine learning algorithms to create new and original content such as images, video, text, audio and even code. There are several types of tools commonly used today:
Chat-based: Utilizing AI-driven conversational agents, such as ChatGPT.
Image-based: Generating images with platforms such as DALL-E or Midjourney.
Music-based: Composing music through AI applications, including Amper Music and AIVA.
Text-based: Producing written content with tools such as Jasper and Writesonic.
Video-based: Creating videos using AI technologies, such as Synthesia and DeepBrain.
Code-based: Assisting in code development with AI tools, including GitHub Copilot.
Software Integration: Enhancing software functionality with AI add-ins, such as Excel or PowerPoint integrations.
Multimodal: Combining multiple modalities of AI, including platforms like ChatGPT, Google DeepMind, and Microsoft Copilot, which integrate various forms of generative AI.
Not sure where to start with AI but want to give it a try? Platforms that you probably use every single day have already integrated generative AI assistants designed to enhance your user experience:
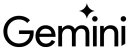
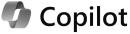

If you use Google, try Google Gemini
If you use Microsoft, try Microsoft Copilot
If you use Facebook or Instagram, try Meta Llama